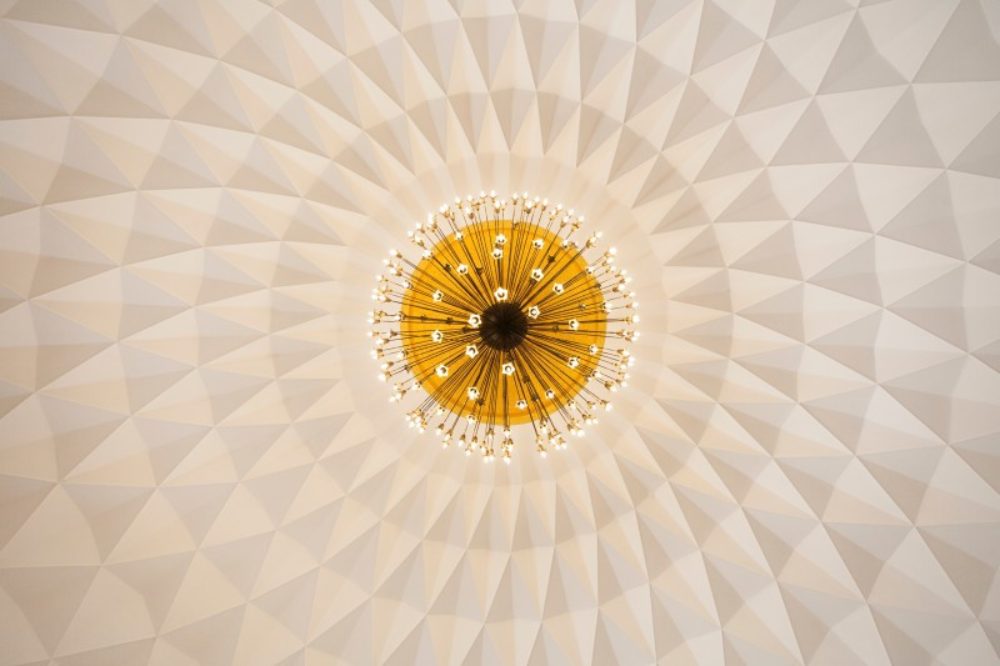
From the early industrial age through the postwar expansion, many of the greatest economic achievements came from bringing together entrepreneurial innovation with the power of standardization, process design and automation. The McCormick harvester and the design and scaling of the McDonald’s franchised restaurant model illustrate the power of this pattern, more than a hundred years apart. Healthcare is perhaps the final major frontier for this paradigm to play out, with Aravind a powerful example of how creative process design, business model design and culture building can yield a level of productivity breathtakingly high above the baseline of an industry.
Another paradigm shift, just as significant in the trajectory of global economic development, is now in its early decades: combining human judgment and the power of algorithms to solve complex problems. In no industry is this work of dancing with the machine more central than in financial services. The frontier of how algorithmic insights and human capabilities can be woven into an effective whole is equally integral to the rarified world of creating alpha as it is to the workaday business of providing customer service.
The Cybernetic Search for Alpha
The idea of the cyborg, an organism that fuses components of biology and machine, will soon celebrate its sixtieth birthday. Hedge funds today are largely cyborg colonies, in which each role in the colony involves a distinct fusion of human and machine work. The lasting creation of alpha isn’t so much in the work of any particular “cyborg” – e.g., an individual trader plus her specific armamentarium of technology – as it is in the building of a larger system in which a whole constellation of human and machine work produces an advantaged pattern of insight generation.
Let’s pull this apart, observing the dance of human and machine first at the level of a trade and then up at the level of the “colony.”
Consider a trader in a long/short equity fund, equipped with the latest AI tools, such as Rumor Hound, which itself uses human experts to seed a machine learning approach to identifying credible signal about mergers and acquisitions from a vast universe of unstructured data. The trader surfaces a rumor that Rumor Hound rates as having high credibility and high market moving potential. She now faces questions of judgment at multiple levels:
- What do I believe about the quality of this signal?
- Beyond this signal, what else do I believe is relevant to assessing this particular opportunity?
- How do I weigh these diverse considerations? Once I’ve come to the resulting synthesis, what actions do I take?
Simply the fact that a human trader can have all kinds of thoughts about these matters of judgment doesn’t in itself establish that the combination of human and machine intelligence will outperform the machine alone. While an above-average human poker player will pick up all sorts of cues that a poker-playing AI cannot, the player may well reduce the quality of the AI’s play by second-guessing its choices. At the same time, in the field of merger arbitrage, it is unlikely that machine-only strategies using commercially available tools will generate alpha for any extended period of time, as these tools become more broadly adopted.
The paragraph above simplifies the dance of human and machine because it isolates the trader contemplating one AI offering one signal, as a prompt for one set of decisions about how to act. While of course there are decisions to be made at this instance level, the more important work is up at the pattern level, about systematization of how signals are combined, confidence levels behind different propositions arrived at, and investment decisions reached.
Now, let’s elevate our focus to the “colony” level of the hedge fund. Up at this level, one steps above the work of specific cybernetic “organisms” – the trader plus her technology tools, combining them by a higher-level algorithm or by a more qualitative feel of the road, and trying to translate insights into alpha – and confronts the sheer difficulty of creating a sustained edge in liquid markets that represent arguably the harshest competitive environment on earth. Just a little bit above the action of the trader are tactical issues of capital deployment: given that the trader is generating positive results over some arc of time, what conclusion can one reach about this, and does it make sense to increase exposure? Important as these calls are, the real action is much further up: what research programs, driven by what people using what tools, approaches and data, have greatest promise to yield pools of alpha that can grow large and that will decay at relatively slow rates? Even if the trading strategies in question are themselves fully machine-driven, judgment about how to build, evolve, deploy and weigh these strategies requires reasoning of a different order. Without making any romantic claims that human beings have permanently superior ability to engage in such higher-level thinking, as a practical matter today, such matters are largely the province of human leaders.
Looking across the landscape of these hedge fund “colonies,” one of the most important forces to observe is the human challenge of assembling the relevant capabilities. This isn’t simply a matter of talent finding – locating (a) the scarce people who bring together the relevant skill sets in machine learning and financial markets; (b) the truly rare people who can shape productive research programs; or even (c) the tiny number of executives who have the combination of market judgment, technical judgment and people judgment to build and steward a “colony.” It’s also the art of how to assemble people who bring different parts of the puzzle – and different blind spots – into effective teams, and how to create a culture that drives the development and interconnection of the right kind of teams. Such cultures are both enabling -- creating intellectual and emotional support for learning and risk-taking -- and challenging: questioning assumptions; revealing points of failure; confronting people with their own blind spots and the blind spots of the cyborgs they create. These questions of individual talent and collective capability are the heart of the battleground, from which the daily tumult of competition in the markets takes its substance and its shape.
Cybernetic Customer Engagement
At the other end of the spectrum of the financial sector from the rarified, secretive pursuit of alpha is the seemingly routine business of engaging and supporting retail customers, from opening an account to collecting on overdue credit. This arena, too, is the work of cyborgs, a frontier for the dance between humans and machines.
Consider the work of credit card collections. After the work of marketing and underwriting has been done, a certain number of accounts become delinquent. Some “cure” quickly, while others drift onward toward the 180-day mark at which the debt must be charged off. As time passes, the probability of repayment drops precipitously, but the value of beating those odds and successfully instituting a payment plan is high. Much of the work here is algorithmic – whom to call and when to call them – but that’s simply a point of entry to the human work of persuading an indebted individual on the other end of the line to pay. At the cybernetic frontier are questions of how machines drive insight into people, and how people and machines team to create the conversation with the highest probability of success.
The most important dimension of human personality for collections is conscientiousness. The reason auto insurers care about FICO scores is that highly conscientious people who guard their credit scores are also safer drivers. One might assume that delinquent card holders have low conscientiousness, but within this pool there’s wide variation. Research has demonstrated that levels of conscientiousness, as well as the other “Big Five” dimensions of personality, can be predicted with reasonable accuracy on the basis of data on an individual’s spending. Now envision the difference between:
(A) the blind, stumbling dance in which an automatic dialer places calls and collection reps pick up those calls that get answered, with no way to distinguish higher- from lower-conscientiousness cardholders
(B) a more tightly choreographed dance in which high-conscientiousness delinquent cardholders are targeted for calls, addressed by collectors in a tone that speaks to someone who is indeed highly conscientious but has likely fallen into difficult circumstances, and given the opportunity to make payment plans that enable them to fulfill their conscientious temperament
Different as the context here is, this represents a parallel fusion of algorithmic insight with human judgment and human touch to what’s required to produce alpha.
Every large retail financial institution manages a support operation that deploys massive resources in a tech system and a human system that together represent the face to their customers. All these institutions are making major investments on the technology side, moving more of their customer engagement into digital channels and harnessing the latest generation of tools to better target and deliver support. Few are investing sufficiently at the intersection of the human system and the tech system, for instance:
- Applying design thinking to understand the customer experiences that human and machine together deliver, and to improve these experiences
- Identifying the frontier of human performance (e.g., how best to convey empathy while resolving a complaint, how best to cross-sell products to specific profiles of customers in particular types of contexts)
- Making that frontier of human performance teachable and scalable, given the power of algorithms to help associates target their approach based on aspects of the customer and their context that wouldn’t be visible to the naked eye
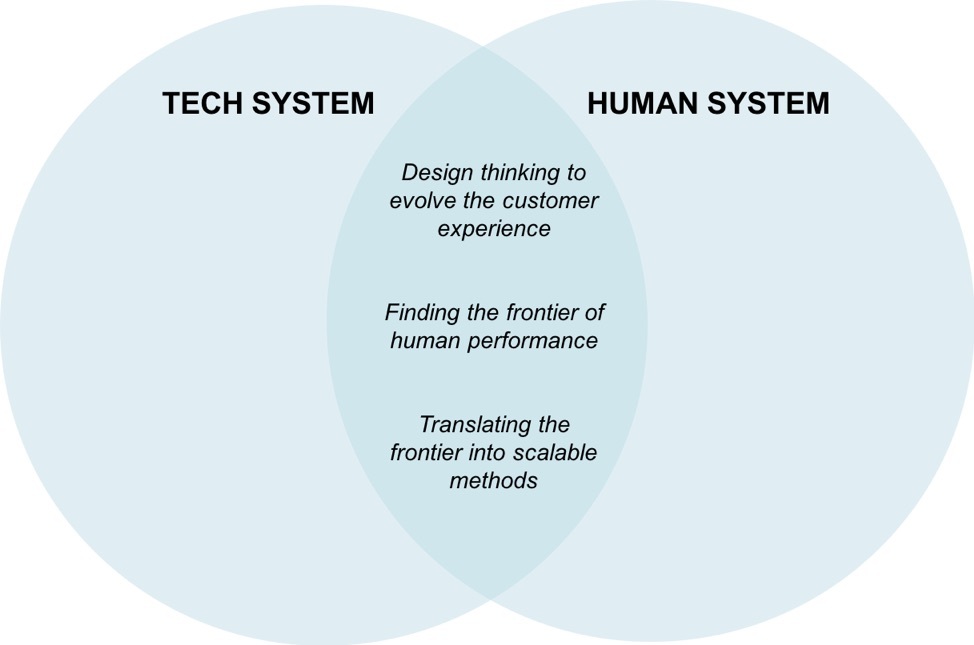
Part of why investment at this intersection of the tech and human systems is chronically too low is that the human systems in operations are run so relentlessly for transactional efficiency. There’s no slack to gather insights about design, develop new approaches, or engage in teaching beyond defined basics. One of the issues here is insufficient division of labor. Even if 98% of the operations workforce needs to be managed to maximize the fraction of “productive time,” small specialized groups can work with the same raw material of customer interaction for the different purposes of innovation, refining and testing techniques, and development of teachable methods – deployed and managed in ways that reflect these objectives rather than transactional productivity metrics. Just as in the domain of alpha production, the gaps here at this critical intersection of the tech and human systems are largely gaps of talent and culture.
Those executives and companies who master the dance of human and machine will establish the critical edge in the next era of financial sector competition. Few CEOs have given this arena proper emphasis. HR executives rarely attend to the immense implications for talent. Technology executives look at only one small part of the equation, even as technology staff become larger and larger fractions of financial sector enterprises. This is perhaps to be expected. It is the same pattern that we’ve seen in production businesses early in the age of automation. But the underlying forces are clear and the future has already arrived. The human work of mastering the dance with the machine is present and graspable, with immense returns to those few who place this work at the center of their focus and keep it there.